In the hospital, we make a lot of important decisions every day, for example about childbirth. Sometimes we do this on the basis of a prediction model: a formula or algorithm. But why do we let something like the choice of treatment depend on a computer instead of a human? And how does a model predict?
Were you born in the hospital? Or maybe your child? There is a good chance that, without you knowing it, a medical algorithm has been used: the APGAR score. The APGAR score is a simple model that determines in about 30 seconds whether newborns appear to be healthy.1 Think of the presence of breathing, movement, heart rate or the color (bluish or not). If the score is low, that is reason to pay extra attention to the baby or intervene immediately. If the score is high, then it seems fine. If necessary, the score will be repeated later. It's so quick to apply and brilliant in its simplicity that most parents don't even realize it! But how is such a score actually developed?
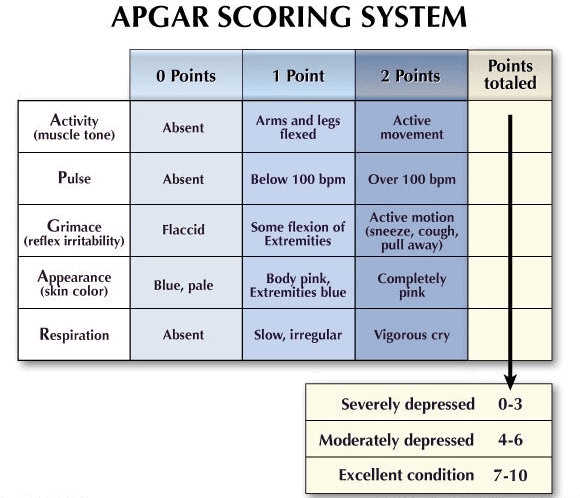
What do you need to be able to predict something?
Actually, you can only predict based on previous observations. These show whether, for example, the bluish baby did indeed need a breathing ventilator later on. Some types of predictions don't look at the past. Take the example of using biomedical knowledge to predict where molecules will bind to see if a new drug can be useful for a particular disease. This involves a completely different type of prediction. However, for most predictions in the world we live in, the basic principle is that real observations must be available. This is called empirical evidence, or data.
Let's say we collect data from babies for a year: their breathing, heart rate, bluish color, and whether treatments were needed later. After that, the math can begin. This is difficult for people. After experiences with many babies, a doctor will probably be able to estimate whether the bluish baby will actually need the ventilator. It gets a lot more complicated when the doctor takes several factors into account: what if the baby has a healthy color, but doesn't move? Or breathes and moves, but is bluish? That makes it a lot more difficult. Also, this judgment is quite subjective: doctors will disagree, which is difficult for the parents to understand in this example. They want a clear decision. Fortunately, the computer offers a solution. Based on statistical models, we can take all these factors into account and weigh them against each other. This is based on the empirical evidence (the data collected earlier), not because one doctor happened to give birth to more bluish babies than another.
Mark van der Wiel uses the same principle when he predicts the rankings of the Top 2000 based on the editions of previous years. Because the art of prediction is of course not limited to the hospital: you can find it everywhere!
Predictive algorithms are everywhere
Well-known applications of prediction models have in common that they are useful to many and easy to interpret, such as the APGAR score. The same goes for the weather! A simple forecast such as the probability that it will rain in the next three hours can save you a wet suit. Even the visualizations of how clouds will move in the near future (for example on the well-known site www.buienradar.nl) are nothing more than distractions of where the clouds are now, plus all the factors that can steer the clouds in certain directions.
Earthquakes are a lot harder to predict. This is due to the complex interplay of many factors that cause an earthquake, which are often difficult or inaccurate to measure.2 Seismic activity is an important part of this story, but by the time it clearly shows that something is wrong, it is probably already too late. An important difference with weather forecasts is that the consequence of the forecast, and therefore also an error in it, can have serious consequences. Let's say the model misses the fact that a severe earthquake will occur in the middle of Tokyo, Japan next week. The damage and suffering will be enormous... So you want to be more sure than when predicting rain. This also applies to a lot of medical research, for example on decisions for fertility treatments, which I have researched (see also my previous blog posts). But more on that later.
A final example is the prediction of stock market prices. When is the best time to invest in stocks? And in which one? Don't be fooled by those who say they can do this: the hard truth is that it's just very difficult to predict.2 The economy is erratic and depends on many factors, many of which cannot even be measured, so you can't put them in the model (for example, whether corona would strike in 2020, or 2019, or 2021). Are you looking for proof? The stock market has many more mild losers than big winners. That will sound familiar to most lottery participants. In addition, the biggest winners are often the people who start with the most money, and can therefore afford very different strategies and margins. See also the hype surrounding GameStop (GME). A chicken-and-gold-and-egg prediction model for the economy does not exist, and may never exist, because its use will affect prices and the economy.
Simplicity vs. Accuracy
What makes a good prediction model? Of course, that depends on what it's intended for. In any case, the result should inform a decision: can the baby go home or should we keep him in the hospital a little longer? Should I wear a raincoat or not? Is now a good time to buy Tesla stock?
Sometimes it is important that it can be applied quickly, where sometimes simplicity is even more important than accuracy. Just think of the APGAR score: after 30 seconds, midwives should know what to do. There's just no time for a grow in the lab.
Sometimes more complex is better, without getting in the way of the intended use. Think about the weather forecast: this data is incredibly complicated, but because it's all processed automatically to make new predictions for tomorrow and the day after, it doesn't really matter. This all takes place 'behind the scenes', the real-time result is now easily usable. The context therefore determines the interpretation and use of prediction models.
So, how do you predict the future? With the past.
It can be immensely helpful to try to predict what's coming based on what has been. The applicability is endless: from the weather to disasters to the hospital (too bad it doesn't really work out in the economy yet). In the hospital it may seem a bit inhumane, this dependence on machines, but the irony is that it is precisely the business and objective of the computer that in many cases produces better predictions than those of doctors or other experts. That's not surprising at all: people make mistakes. And given the consequences of making a wrong diagnosis or starting the wrong treatment, we prefer to limit that. Then just carry out what the computer advises. Although I prefer not to let my financial decisions depend on that...
Sources:
- APGAR score: see for example https://www.youtube.com/watch?v=98ikG8a9JKs&ab_channel=Medzcool.
- The Signal and the Noise, boek over voorspelmodellen geschreven door Nate Silver.